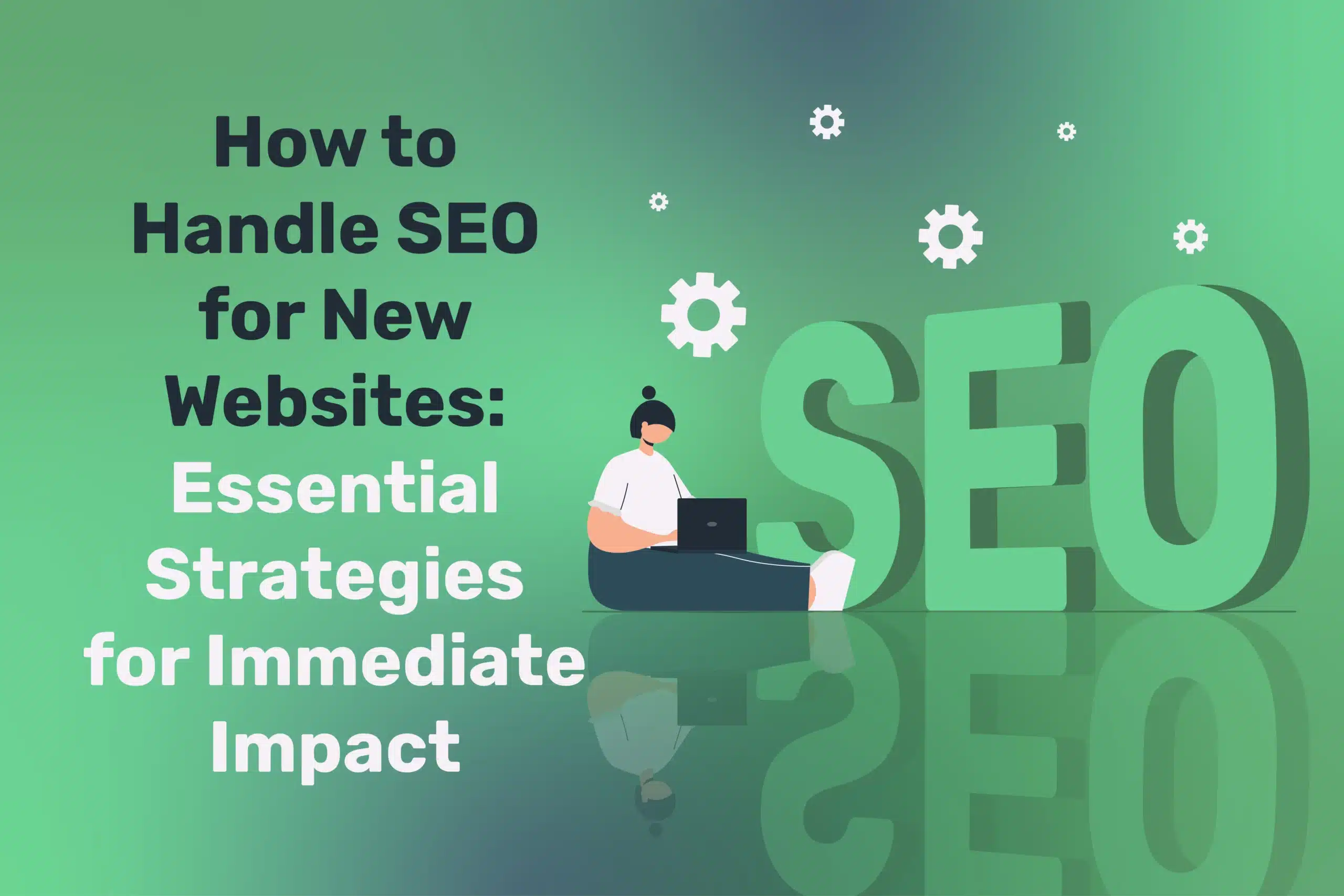
Analytics in AI Adoption: A Data-Driven Approach for UK and Irish SMEs
Table of Contents
Many small to medium-sized enterprises (SMEs) across Ireland, Northern Ireland, and the UK launch AI initiatives—such as chatbots or machine learning analytics—only to face challenges in measuring concrete returns or guiding strategic improvements. Data analytics offers the solution, providing essential feedback that clarifies whether AI implementations are meeting business objectives or require recalibration. This comprehensive guide explains how businesses can effectively utilise web analytics, user metrics, and AI performance data to optimise their AI tools, maximising return on investment and securing long-term viability.
“Installing AI is just the first step. Without consistent analytics to see what’s working, you risk operating on assumptions rather than facts. A data-driven approach guarantees your AI aligns with actual business requirements,” says Ciaran Connolly, Director of ProfileTree.
Why Analytics in AI Adoption Matter
Analytics play a crucial role in AI adoption by providing data-driven insights that optimise performance, measure impact, and ensure responsible deployment. By tracking key metrics like accuracy, efficiency, and user engagement, businesses can refine AI models, enhance decision-making, and demonstrate ROI, leading to more effective and ethical AI integration.
Validating ROI
With average AI implementation costs reaching £9,500 for small UK businesses, owners need clear visibility into whether chatbots genuinely reduce support costs or if advanced machine learning delivers more accurate product forecasting. Analytics uncovers these cost savings and performance improvements with precision.
Identifying Improvement Areas
If your Belfast-based support chatbot struggles with Gaelic queries, analytics might reveal high abandonment rates or patterns of unanswered questions. These data points signal when to fix or retrain your model specifically for local language inputs, enhancing user experience for your regional audience.
Minimising Risks
AI implementations can potentially operate as “black box” decision-makers. By tracking each step—such as AI-based loan recommendations—analytics can highlight anomalies or biases. Detecting these issues early prevents reputational damage and compliance issues under increasingly stringent UK and EU regulations.
Key Metrics for AI Projects
Tracking the right metrics is essential for evaluating AI project success. Key performance indicators (KPIs) include model accuracy, response time, user engagement, and cost efficiency. Additionally, monitoring bias, data quality, and compliance ensures ethical AI deployment. Regular analysis of these metrics helps refine AI models and maximise business impact.
Chatbot-Related Analytics
- Deflection Rate: The percentage of user queries successfully resolved by the chatbot without human intervention.
- User Satisfaction: Measured through immediate feedback mechanisms like star ratings or thumbs up/down options within the chat interface.
- Common Fallback Queries: Recurring user requests that the chatbot cannot handle—revealing gaps in training data that require attention.
ML Model Accuracy
For demand forecasting or fraud detection systems, critical measurements include:
- Precision/Recall: Are you accurately identifying genuine fraud cases while minimising false positives?
- RMSE (Root Mean Square Error) or MAPE (Mean Absolute Percentage Error) for forecasting models: Quantifies the difference between predicted versus actual sales or inventory usage.
- Confusion Matrix Analysis: Detailed breakdown of true positives, false positives, true negatives, and false negatives to identify specific improvement areas.
Time/Cost Savings
Track staff hours previously dedicated to manual tasks (such as data entry) that have been automated. For example, if a small Cork-based accounting firm saves 12 hours weekly through automation, that represents quantifiable ROI directly linked to AI implementation logs or time-tracking systems.
Select metrics that directly connect to your business objectives, not merely arbitrary AI statistics. Whether you’re tracking escalation rates or calculated cost savings, define success criteria from the outset,” advises Ciaran Connolly.
Gathering Data: Tools and Techniques
Effective AI adoption relies on high-quality data, making the right tools and techniques essential. Businesses can use data collection platforms, APIs, and analytics tools like Google Cloud AI or AWS SageMaker to gather and process information. Techniques such as web scraping, surveys, and real-time monitoring help ensure diverse, accurate, and unbiased datasets for AI training and optimisation.
Web Analytics (Google Analytics 4, etc.)
- User Journeys: For website-based AI chatbots, track how many sessions begin or conclude with bot interaction, changes in time on page, and shifts in bounce rates.
- Conversion Goals: Configure AI interactions as micro-conversions (such as users completing a chatbot support flow or generating a qualified sales lead).
- Event Tracking: Monitor specific interactions with AI tools, including button clicks, question types, and resolution pathways.
AI-Specific Dashboards
Vendors or custom development teams often provide AI consoles displaying model usage statistics, error rates, or training progression metrics. Advanced solutions may offer “model drift” alerts when real-world data significantly diverges from training assumptions, prompting timely intervention.
Heatmaps and Session Recordings
Tools such as Hotjar or Microsoft Clarity can reveal whether visitors actively engage with your chatbot widget or bypass it entirely. For physical retail applications (like kiosks with AI-based product recommendations), analytics might incorporate hardware logs or footfall data from CCTV systems to measure engagement levels.
Customer Feedback Systems
Direct feedback through surveys, support tickets, and customer interviews provides qualitative context to quantitative metrics, revealing sentiment and satisfaction levels that raw numbers might miss.
Using Analytics to Refine Chatbots
Analytics help optimise chatbots by tracking key metrics like response accuracy, user satisfaction, and engagement rates. By analysing conversation logs, identifying drop-off points, and monitoring sentiment, businesses can refine chatbot responses and improve user experience. Continuous testing and AI model adjustments ensure chatbots become more intuitive, efficient, and aligned with customer needs.
Identify Unanswered Queries
Compile all queries triggering fallback responses or “I don’t understand” messages. Group these by topic or intent. If numerous queries relate to “store returns in Northern Ireland,” expand your knowledge base with relevant policy information or retrain the model accordingly.
Monitor Engagement Over Time
If initial usage statistics are high but subsequently decline, your chatbot may have lost its novelty appeal or might be providing overly generic answers. For instance, Irish users might prefer Gaelic greetings. Consider localising content or adding conversational capabilities that reflect your brand personality and regional understanding.
A/B Testing
Implement controlled tests comparing different chatbot variants. One version might greet users with “How can we help you today, from anywhere in the UK or Ireland?” while another automatically presents popular topic options. Compare user satisfaction rates, time to resolution, and conversion metrics to determine optimal configurations.
Natural Language Processing (NLP) Refinement
Analyse conversation logs to identify misinterpreted phrases, regional terminology, or industry jargon that confuses your AI. Use these insights to enhance your NLP models with specialised vocabularies relevant to UK and Irish markets.
Refining Machine Learning (ML) Models with Analytics
Analytics play a vital role in improving machine learning models by identifying patterns, detecting biases, and optimising performance. Key metrics like precision, recall, and F1-score help assess accuracy, while A/B testing and real-time feedback loops enable continuous refinement. By leveraging data insights, businesses can enhance model reliability, efficiency, and real-world applicability.
Regular Performance Reviews
Schedule monthly or quarterly audits comparing ML model predictions against actual outcomes. If higher error rates appear in specific user segments (such as rural locations or certain Gaelic-labelled addresses), retrain the model with augmented datasets targeting these weak points.
Feedback Loops
Establish mechanisms for staff or users to flag incorrect outcomes. For example, if your system incorrectly identifies a transaction as fraudulent, enable employees to mark it as a “false positive.” These labels feed back into the training dataset to reduce similar errors in future. Over time, this creates a self-improving system.
Rolling Updates
Instead of major annual overhauls, implement continuous training approaches for systems with regular data flows. This keeps models current and responsive, particularly important in dynamic markets characterised by seasonal shifts (such as retail cycles or regional travel patterns).
Feature Importance Analysis
Regularly evaluate which data inputs most significantly influence your ML model’s decisions. This helps identify critical variables while potentially reducing computational complexity by eliminating low-impact factors.
“ML models are never truly complete. Ongoing feedback from both staff and systematic data logs continuously refines them, maintaining consistent accuracy even as market conditions evolve,” says Ciaran Connolly.
E-E-A-T and Helpful Content for AI Tools
Ensuring AI tools align with E-E-A-T (Experience, Expertise, Authoritativeness, and Trustworthiness) enhances credibility and user trust. Providing transparent documentation, expert-driven insights, and real-world case studies strengthens their reliability. AI-generated content should prioritise accuracy, ethical data use, and user value to meet search quality standards and drive meaningful engagement.
Transparent Knowledge Base
For customer-facing AI solutions on your website (such as recommendation engines), provide clear documentation explaining functionality, data usage policies, and potential regional variations. This demonstrates Experience and Expertise to users, fulfilling Google’s “helpful content” guidelines.
Authoritative Documentation
In developer blogs or user manuals for your AI tools, include references and authentic case studies. Showcase behind-the-scenes processes and local staff involvement in development. This approach builds trust through transparency.
Trust Through Clear Disclaimers
When AI-based recommendations cannot guarantee 100% accuracy, include appropriate disclaimers. For example: “Recommended items are based on your shopping patterns—final stock availability may vary by location.” This honest approach reinforces the “Trust” dimension of E-E-A-T, reassuring users about limitations.
Accessibility Considerations
Ensure AI tools adhere to accessibility standards, with clear documentation on how your systems accommodate diverse user needs across UK and Irish markets, further enhancing trust and expertise signals.
Integrating Analytics with AI Tools: A Step-by-Step Implementation Guide
- Define AI Objectives: Establish concrete goals such as reducing support time by 30% or improving sales forecasting accuracy by 15% for your UK market.
- Select Relevant Metrics: Identify key indicators including chatbot deflection rate, ML error margins, staff hour savings, and user satisfaction scores that align with your objectives.
- Set Up Tracking Infrastructure: Configure GA4 events for chatbot interactions, implement AI monitoring consoles for ML logs, or develop custom dashboards integrating data from your regional CRM.
- Initial Pilot Phase: Collect baseline metrics over 1–2 months to identify quick wins and critical issues requiring immediate attention.
- Refinement Process: Retrain models or enhance chatbot scripts where data indicates performance gaps. Optimise user prompts and add regionally relevant references to improve engagement.
- Ongoing Monitoring Protocol: Establish weekly or monthly KPI reviews. When specific metrics decline, investigate root causes—such as staff turnover or seasonal data variations.
- Strategic Expansion: Once success is demonstrated, incrementally add enhanced AI functionalities or deeper analytical capabilities based on proven performance.
Define success metrics at project initiation—whether that’s time saved or forecast accuracy improvements. Then continuously measure, refine, and scale your implementation. This iterative approach forms the foundation of effective AI strategy,” advises Ciaran Connolly.
Common Pitfalls and Practical Solutions
AI adoption comes with challenges like data bias, inaccurate predictions, and poor user experience. Ignoring proper model training, lacking transparency, or failing to monitor performance can lead to unreliable results. Practical solutions include continuous data refinement, ethical AI practices, and using analytics to track and improve model effectiveness over time.
Data Overload
Many organisations struggle with excessive logs and analytics without extracting actionable insights. Focus on a select group of meaningful metrics. Provide management with monthly insight summaries highlighting key changes and recommended next steps.
Staff Resistance
When employees feel threatened by AI or question the relevance of usage statistics, targeted internal training becomes essential. Demonstrate how analytics helps them perform more effectively—enabling them to focus on complex customer interactions while automation handles routine enquiries.
Biased Interpretation
Decision-makers sometimes interpret data selectively to confirm existing biases. Maintain objective analysis methodologies, cross-referencing with staff feedback and user comments. If data indicates your chatbot is underperforming for Gaelic speakers, address this reality constructively rather than dismissing inconvenient findings.
Incomplete Integration
Siloed analytics systems often fail to provide comprehensive insights. Implement integrated dashboards that combine AI performance metrics with broader business KPIs to reveal correlations and causal relationships.
Future Outlook: Enhanced AI with Sophisticated Analytics
As AI evolves, sophisticated analytics will play a crucial role in improving accuracy, efficiency, and ethical deployment. Advanced techniques like real-time monitoring, predictive modelling, and automated anomaly detection will refine AI performance. Businesses leveraging these insights will drive smarter decision-making, more personalised user experiences, and greater overall AI reliability.
Real-Time AI Performance Monitoring
As SMEs adopt increasingly advanced tools, real-time monitoring dashboards will become standard. Intelligent alert systems for anomaly detection (such as “Our chatbot’s fallback responses increased by 40% this morning for Cork-based queries”) will enable immediate intervention by support teams.
Voice and Augmented Reality Data Integration
With voice search and AR becoming mainstream components of user experiences, analytics frameworks must expand to track these interaction methods. For example, understanding why “Chatbot usage increased among Gaelic speakers in Donegal” might inform content strategy adjustments to better serve specific regional markets.
AI-Driven Personalisation Refinement
Analytics will increasingly guide personalisation capabilities. If data reveals Northern Ireland customers prefer specific delivery timeframes, your AI could automatically suggest these options for Belfast or Derry addresses. These micro-optimisations compound over time to significantly enhance customer satisfaction.
Predictive Analytics for AI Optimisation
Next-generation systems will anticipate when AI tools require retraining or enhancement before performance degradation occurs, based on early warning signals identified through sophisticated pattern recognition.
Creating a Continuous Cycle of Data-Driven AI Improvement
Implementing AI represents only half the journey—analytics completes the process by revealing whether chatbots genuinely reduce support volumes or if forecasting models accurately predict sales for your regional operations in locations like Cork or Belfast. By monitoring essential KPIs (including deflection rates, model accuracy, and quantifiable cost savings) and integrating them with comprehensive web analytics (such as session duration and user journeys), your organisation guarantees AI alignment with concrete business objectives.
Consistently refining AI solutions based on data-driven insights not only drives immediate ROI but strengthens your brand’s E-E-A-T credentials: demonstrating continuous domain experience, expert handling of customer requirements, and a trustworthy approach to transparent improvement processes. For SMEs across Ireland, Northern Ireland, and the broader UK market, this synergy between AI implementation and analytics creates a foundation for sustainable, forward-thinking success in an increasingly competitive digital landscape.
“Post-deployment analytics serve as the true engine of AI success. Listen to what your data reveals, systematically address blind spots, and watch your AI transform from an interesting novelty into a genuine business accelerator,” concludes Ciaran Connolly.
Getting Started with AI Analytics at Your Organisation
Implementing AI analytics in your organisation starts with defining clear objectives and selecting the right tools. Identify key metrics, ensure high-quality data, and leverage platforms like Google Cloud AI or Azure Machine Learning. Begin with small pilot projects, analyse results, and refine strategies to scale AI adoption effectively.
Conduct an AI Readiness Assessment
Before diving into complex analytics, evaluate your current data infrastructure and team capabilities. Many UK and Irish SMEs discover existing systems require modernisation before supporting sophisticated AI analytics.
Start Small, Scale Strategically
Begin with a focused AI project addressing a specific business challenge, accompanied by clearly defined metrics. This approach allows for manageable implementation and measurable success before expanding to broader applications.
Build Cross-Functional Teams
Effective AI analytics requires collaboration between technical specialists and business domain experts. Create teams that combine data scientists with staff who possess deep understanding of regional market dynamics across Ireland and the UK.
Develop an AI Analytics Roadmap
Chart a progressive implementation timeline spanning 12-24 months, aligning AI initiatives with broader digital transformation goals while accounting for potential regulatory changes affecting UK and Irish markets.
How ProfileTree Supports Data-Driven AI Implementation
ProfileTree specialises in helping UK and Irish SMEs implement effective, analytics-driven AI solutions tailored to regional market requirements. Our comprehensive approach includes:
- AI Readiness Assessments: Evaluating your current digital infrastructure and identifying preparation requirements for successful implementation.
- Custom Analytics Dashboards: Developing bespoke monitoring systems that track AI performance against your specific business objectives.
- Ongoing Optimisation Services: Providing continuous support to refine AI tools based on performance data and changing business needs.
- Staff Training Programmes: Equipping your team with the skills to interpret AI analytics and implement data-driven improvements.
By partnering with local UK and Irish businesses to implement analytics-focused AI strategies, ProfileTree delivers solutions that drive measurable ROI while adapting to the unique requirements of regional markets.