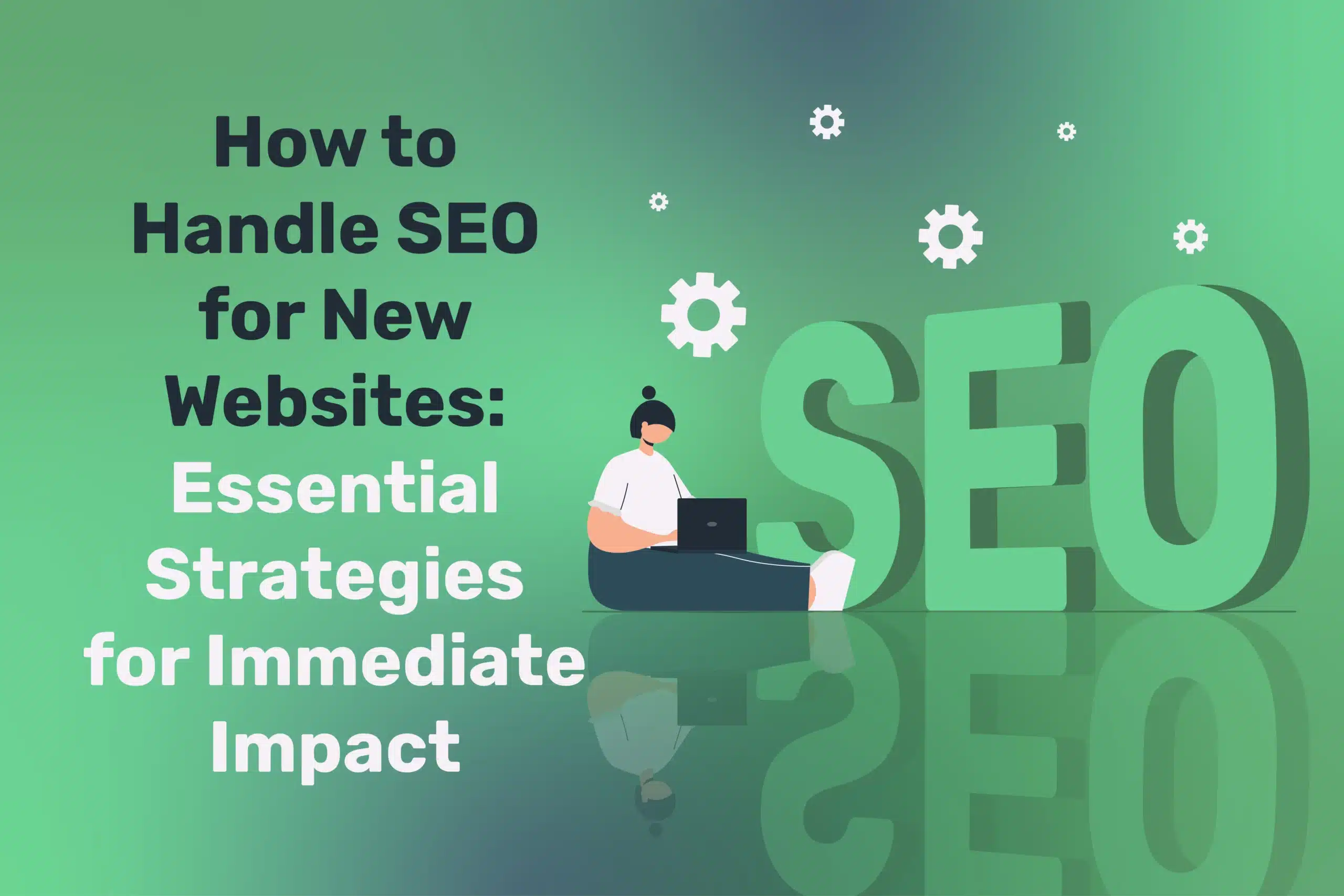
Leveraging AI for Business Success: Transformative Tools and Strategies for the Modern Enterprise
Table of Contents
In today’s rapidly evolving digital landscape, artificial intelligence tools have become indispensable for businesses seeking enhanced efficiency, significant cost reductions, and sustainable competitive advantages. From sophisticated chatbots managing customer support to Robotic Process Automation (RPA) streamlining repetitive tasks, AI adoption enables organisations to automate complex workflows, extract valuable insights from large datasets, and deliver personalised customer experiences. As of 2024, approximately 70% of mid-sized companies have implemented at least one AI solution, a substantial increase from 40% in 2022—highlighting the accelerating adoption of these transformative technologies.
This comprehensive guide explores the most impactful AI categories for business applications, detailing their functionality, benefits, implementation strategies, and potential challenges. Whether you operate a startup, small to medium enterprise, or large corporation, understanding these tools can substantially accelerate your digital transformation initiatives while ensuring measurable return on investment.
Why AI Tools Are Essential for Modern Businesses
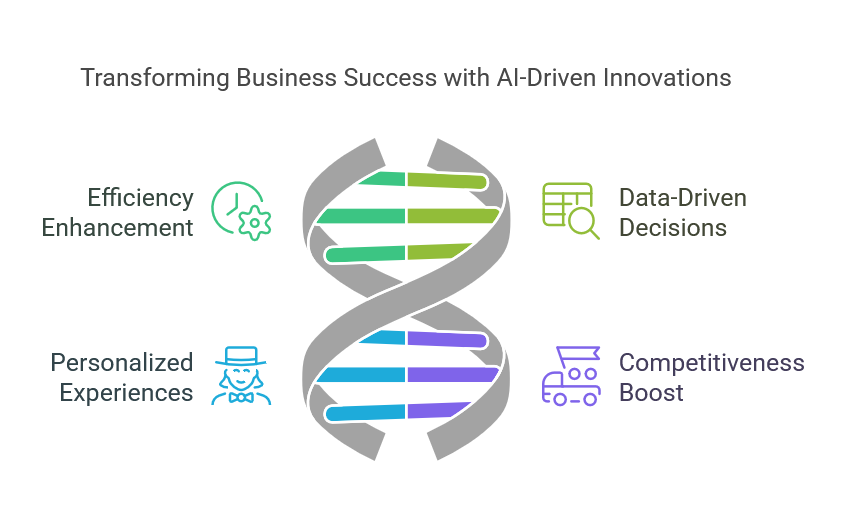
AI tools are crucial for modern businesses. They enhance efficiency, drive data-driven decisions, and deliver personalised customer experiences, all while boosting competitiveness.
Efficiency and Productivity Enhancements
- Time Optimisation: Automating labour-intensive tasks—such as data entry, document processing, and routine communications—liberates valuable staff time for higher-value activities requiring creative thinking and strategic decision-making.
- Resource Allocation: Implementing chatbots, automated workflow systems, and scheduling assistants provides continuous operational capability without corresponding increases in staffing costs or burnout risks.
- Process Standardisation: AI-driven automation ensures consistent application of business rules and procedures, reducing error rates and improving quality control across operations.
Data-Driven Decision Making
- Advanced Analytics: AI solutions can process enormous datasets—millions of transactions, customer interactions, or operational metrics—identifying patterns, anomalies, and opportunities invisible to traditional analysis methods.
- Predictive Capabilities: Machine learning models enable proactive business strategies through demand forecasting, customer churn prediction, maintenance scheduling, and inventory optimisation.
- Real-Time Intelligence: Modern AI platforms deliver continuous data analysis and insights, allowing businesses to respond rapidly to market changes, emerging trends, or operational issues.
Enhanced Customer Experiences
- Personalisation: AI-powered systems create tailored experiences by analysing individual preferences, purchase history, and behavioural patterns to deliver relevant content, product recommendations, and service offerings.
- 24/7 Engagement: Intelligent virtual assistants and chatbots respond immediately to customer enquiries regardless of time zones or business hours, significantly improving satisfaction and conversion rates.
- Journey Optimisation: AI tools analyse customer interactions across multiple touchpoints, identifying friction points and opportunities to streamline the customer journey.
Competitive Advantage
- Innovation Acceleration: AI enables rapid testing and refinement of new products, services, and business models through simulation, market analysis, and customer feedback processing.
- Operational Agility: Advanced predictive capabilities help businesses anticipate market shifts and customer needs, allowing for proactive strategy adjustments rather than reactive responses.
- Scalability: AI-powered solutions often scale more efficiently than traditional systems, enabling businesses to expand operations without proportional increases in resources or costs.
Key AI Tool Categories for Business Applications
Explore the key AI tool categories transforming business operations, from automation and analytics to customer engagement and content generation.
Conversational AI and Chatbots
Leading Solutions: ChatGPT, Claude, Google Bard, Watson Assistant, Drift, Intercom
Primary Applications:
- Customer Support: Automatically address frequently asked questions, troubleshoot common issues, and route complex enquiries to appropriate human specialists
- Sales Enablement: Engage website visitors, qualify leads, answer product questions, and facilitate purchasing decisions
- Internal Knowledge Management: Provide employees with immediate access to company information, policies, and procedures
Implementation Guidance: Effective chatbot deployment requires careful planning and ongoing refinement:
- Develop a comprehensive knowledge base covering common customer questions and scenarios
- Create clear escalation pathways for complex issues requiring human intervention
- Continuously analyse conversation logs to identify improvement opportunities
- Incorporate personality elements aligned with your brand voice and values
Success Measurement: Track metrics, including resolution, customer satisfaction scores, conversation completion rates, and support ticket reduction.
Robotic Process Automation (RPA)
Leading Solutions: UiPath, Automation Anywhere, Blue Prism, Microsoft Power Automate
Primary Applications:
- Financial Operations: Automate invoice processing, payment reconciliation, expense management, and financial reporting
- Human Resources: Streamline employee onboarding, timesheet processing, leave management, and benefits administration
- Supply Chain Management: Automate purchase order creation, inventory updates, supplier communications, and logistics coordination
Implementation Guidance: Successful RPA deployment requires:
- Process mapping to identify high-volume, rule-based activities suitable for automation
- Calculating potential ROI based on time savings, error reduction, and staff reallocation
- Starting with contained projects demonstrating quick wins before broader implementation
- Establishing governance procedures for bot management, monitoring, and maintenance
Success Measurement: Monitor automation rate, error reduction, processing time improvements, cost savings, and employee satisfaction with new workflows.
Advanced Analytics and Predictive Tools
Leading Solutions: Google Vertex AI, Microsoft Azure Machine Learning, Dataiku, DataRobot, Amazon SageMaker
Primary Applications:
- Demand Forecasting: Predict product demand across different markets, seasons, and customer segments to optimise inventory and production planning
- Customer Analytics: Identify churn risk, lifetime value potential, and next-best-action recommendations for individual customers
- Operational Optimisation: Predict maintenance needs, resource requirements, and potential bottlenecks before they impact business performance
Implementation Guidance: Effective analytics implementation requires:
- Establishing data governance practices ensuring quality, accessibility, and compliance
- Developing clear business questions that analytics should address
- Creating cross-functional teams combining domain expertise with technical capabilities
- Implementing validation procedures to ensure model accuracy and reliability
Success Measurement: Evaluate prediction accuracy, business impact of insights, decision-making speed, and quantifiable outcomes from data-driven initiatives.
Content Generation and Management
Leading Solutions: Jasper.ai, Copy.ai, Writesonic, Canva AI, Grammarly Business
Primary Applications:
- Marketing Content: Generate social media posts, email campaigns, ad copy, and product descriptions aligned with brand guidelines
- Content Strategy: Develop topic ideas, content outlines, and SEO recommendations based on market trends and competitor analysis
- Communications: Draft internal announcements, meeting summaries, and standard business correspondence
Implementation Guidance: Responsible content AI usage includes:
- Establishing clear editorial guidelines for AI-assisted content creation
- Implementing human review processes before publication
- Training AI systems with company-specific examples and terminology
- Ensuring content aligns with brand voice, expertise standards, and accuracy requirements
Success Measurement: Track content production efficiency, engagement metrics, conversion rates, and channel quality consistency.
Marketing Automation and Personalisation
Leading Solutions: Marketo Engage, HubSpot Marketing Hub, Salesforce Marketing Cloud, Klaviyo, Omnisend
Primary Applications:
- Customer Segmentation: Dynamically group customers based on behaviour, preferences, and lifecycle stage for targeted marketing
- Omnichannel Campaigns: Coordinate personalised messaging across email, social media, SMS, and web channels
- Customer Journey Optimisation: Create adaptive marketing sequences responding to individual engagement patterns
Implementation Guidance: Effective marketing automation requires:
- Developing detailed customer personas and journey maps
- Integrating data sources for comprehensive customer profiles
- Creating decision rules and content variants for different segments
- Establishing testing protocols to optimise campaign performance
Success Measurement: Monitor engagement rates, conversion improvements, customer lifetime value changes, and marketing ROI enhancements.
Computer Vision and Image Recognition
Leading Solutions: Google Cloud Vision API, Amazon Rekognition, Microsoft Computer Vision, IBM Watson Visual Recognition
Primary Applications:
- Quality Control: Automatically inspect products or components for defects with greater consistency than human inspection
- Security and Safety: Monitor facilities for safety compliance, unauthorised access, or potential hazards
- Retail Analytics: Track customer movement patterns, shelf inventory levels, and product placement effectiveness
Implementation Guidance: Successful computer vision deployment includes:
- Building comprehensive image training datasets representing all scenarios
- Starting with contained use cases before expanding to more complex applications
- Implementing appropriate privacy safeguards and notifications
- Creating exception-handling procedures when confidence thresholds aren’t met
Success Measurement: Evaluate detection accuracy, false positive/negative rates, processing speed, and business impact metrics relevant to specific applications.
Strategic Implementation Framework for AI Adoption
A strategic framework for AI adoption ensures businesses successfully integrate AI tools, align with objectives, optimise data infrastructure, and drive long-term success.
Establishing Clear Business Objectives
Before selecting specific AI tools, organisations should:
- Identify specific business challenges or opportunities AI could address
- Define measurable success criteria and expected outcomes
- Prioritise potential applications based on business impact and implementation feasibility
- Align AI initiatives with broader strategic objectives and digital transformation roadmaps
Data Infrastructure and Readiness
AI systems require quality data foundations:
- Conduct data audits to assess the availability, quality, and accessibility of required information.
- Implement data governance frameworks ensuring compliance with privacy regulations.
- Develop data cleaning and preparation protocols appropriate for AI applications.
- Create data integration strategies connecting relevant systems and information sources.
Phased Implementation Approach
A staged deployment strategy maximises success probability:
- Begin with pilot projects addressing well-defined, high-impact use cases
- Establish clear evaluation criteria and measurement frameworks
- Document learnings and refine approaches before expanding the scope
- Develop internal success stories to build organisational support for broader adoption
Organisational Change Management
AI implementation represents significant operational change:
- Communicate clear rationale and benefits to all stakeholders
- Provide comprehensive training for employees using or affected by AI systems
- Address concerns about job displacement by emphasising augmentation rather than replacement
- Celebrate early successes and share positive outcomes throughout the organisation
Ongoing Optimisation and Governance
AI systems require continuous management:
- Establish monitoring protocols for system performance and business impact
- Implement regular review cycles to assess accuracy, relevance, and effectiveness
- Create feedback mechanisms for users to report issues or suggest improvements
- Develop governance frameworks addressing ethical considerations, bias mitigation, and compliance requirements
Common Implementation Challenges and Mitigation Strategies
Implementing AI involves data security, integration issues, and skill gaps. This section explores common obstacles and strategies for effectively overcoming them.
Balancing Automation and Human Oversight
Challenge: Over-reliance on AI systems without appropriate human supervision can lead to errors, customer dissatisfaction, or compliance issues.
Mitigation Strategies:
- Implement confidence thresholds triggering human review for uncertain cases.
- Create clear escalation pathways for complex scenarios
- Design hybrid workflows combining AI efficiency with human judgment
- Regularly audit automated decisions to identify improvement opportunities
Data Security and Privacy Concerns
Challenge: AI systems often process sensitive information, creating potential risks around data protection, privacy compliance, and security vulnerabilities.
Mitigation Strategies:
- Implement comprehensive data protection protocols, including encryption and access controls.
- Ensure compliance with relevant regulations (GDPR, CCPA, industry-specific requirements)
- Create transparent data usage policies and communicate them clearly to customers.
- Conduct regular security assessments of AI implementations and data workflow.s
Integration with Legacy Systems
Challenge: Many organisations struggle to connect AI tools with existing technology infrastructure, limiting effectiveness and creating information silos.
Mitigation Strategies:
- Develop comprehensive integration strategies before implementation.
- Consider API-first solutions designed for enterprise connectivity
- Implement middleware solutions where direct integration isn’t feasible
- Create data synchronisation protocols ensuring consistency across systems
Skill Gaps and Resource Limitations
Challenge: Effective AI implementation requires specialised knowledge that many organisations lack internally.
Mitigation Strategies:
- Develop targeted training programmes for existing staff
- Consider strategic hiring to address critical skill gaps
- Explore partnerships with specialised consultancies or solution providers
- Leverage no-code/low-code AI platforms requiring less technical expertise
Maintaining System Relevance and Accuracy
Challenge: AI systems require ongoing maintenance as business requirements, customer behaviours, and market conditions evolve.
Mitigation Strategies:
- Schedule regular model retraining and performance evaluations
- Monitor data drift indicating changes in underlying patterns
- Implement feedback loops capturing system performance issues
- Create clear ownership and responsibility for ongoing maintenance
Emerging Trends Shaping the Future of Business AI (2024 and Beyond)
As AI evolves, new trends are reshaping business applications. This section explores the emerging innovations and technologies that will define the future of AI in business.
Multimodal AI Systems
Future business AI will seamlessly integrate multiple data types:
- Combined text, image, audio, and video processing capabilities
- Unified interfaces for diverse AI applications
- Cross-modal learning improving overall system intelligence
- Enhanced ability to understand complex business contexts and scenarios
Emotionally Intelligent Business Systems
Next-generation AI will incorporate emotional intelligence:
- Advanced sentiment analysis detecting customer feelings and intentions
- Adaptive communication adjusting tone and approach based on emotional context
- Empathetic chatbots provide more natural customer interactions
- Feedback systems capturing emotional responses to business communications
Democratised AI Development
AI capabilities will become accessible to non-technical business users:
- No-code platforms enabling business analysts to create custom AI solutions
- Automated machine learning streamlining model development and deployment
- Pre-built industry-specific solutions requiring minimal customisation
- Self-service analytics with built-in AI capabilities for business teams
Enhanced Privacy-Preserving Technologies
New approaches will address data privacy concerns:
- Federated learning enabling model training without centralising sensitive data
- Synthetic data generation for testing and development without privacy risks
- Differential privacy techniques protecting individual information while maintaining analytical value
- Privacy-by-design frameworks embedded in AI development methodologies
Autonomous Decision Systems
Advanced AI will increasingly operate with greater autonomy:
- Self-optimising marketing systems adjusting campaigns without human intervention
- Automated financial controls responding to market conditions in real-time
- Supply chain systems autonomously adapt to disruptions or demand changes
- Predictive maintenance systems scheduling interventions based on equipment conditions
Building an AI-Ready Organisation
Building an AI-ready organisation involves fostering a data-driven culture, developing cross-functional expertise, and preparing your team to leverage AI for sustained growth and innovation.
Creating a Data-Driven Culture
Successful AI implementation requires organisational readiness:
- Establish data literacy programmes across all levels of the organisation
- Incentivise data-driven decision-making rather than intuition alone
- Create accessible dashboards and visualisations, making insights widely available
- Celebrate successes resulting from data-informed strategies
Developing Cross-Functional Expertise
AI thrives at the intersection of technical and domain knowledge:
- Create collaborative teams combining subject matter experts with technical specialists
- Develop shared vocabulary and understanding across business and technical functions
- Implement knowledge-sharing mechanisms to disseminate insights
- Recognise and reward cross-functional collaboration on AI initiatives
Ethical AI Framework Development
Responsible AI implementation requires ethical guardrails:
- Establish principles governing AI development and deployment
- Create review processes for identifying potential bias or unintended consequences
- Implement transparency practices explaining how AI systems make decisions
- Develop monitoring systems ensuring ongoing compliance with ethical standards
Strategic Technology Partnerships
Few organisations possess all required AI capabilities internally:
- Assess potential technology and implementation partners based on industry expertise
- Consider the ecosystem surrounding potential AI solutions
- Evaluate support capabilities and long-term viability of providers
- Develop balanced relationships, maintaining organisational control while leveraging external expertise
Industry-Specific AI Applications and Case Studies
This section highlights industry-specific AI applications and real-world case studies, demonstrating how businesses across sectors successfully leverage AI to drive growth and efficiency.
Retail and E-commerce
Transformative Applications:
- Inventory optimisation through predictive demand forecasting
- Personalised shopping experiences based on individual preferences
- Visual search enabling product discovery from images
- Dynamic pricing adjusting to market conditions and consumer behaviour
Implementation Example: A mid-sized fashion retailer implemented AI-powered inventory management, reducing stockouts by 32% while decreasing excess inventory by 28%, resulting in significant margin improvements.
Financial Services
Transformative Applications:
- Fraud detection identifying suspicious transactions in real-time
- Automated underwriting streamlining loan approval processes
- Personalised financial advice based on individual circumstances
- Compliance monitoring ensures regulatory adherence
Implementation Example: A regional bank deployed AI-powered fraud detection, reducing false positives by 47% while increasing actual fraud identification by 23%, significantly improving customer experience and reducing losses.
Manufacturing
Transformative Applications:
- Predictive maintenance prevents equipment failures before they occur
- Quality control using computer vision for consistent inspection
- Supply chain optimisation anticipating disruptions and adjusting accordingly
- Energy usage optimisation reduces costs and environmental impact
Implementation Example: A manufacturing facility implemented AI-based predictive maintenance, reducing unplanned downtime by 37% and extending equipment lifespan by an average of 23%, delivering substantial operational and financial benefits.
Healthcare and Life Sciences
Transformative Applications:
- Diagnostic assistance improving accuracy and speed
- Treatment plan optimisation based on patient-specific factors
- Clinical trial matching identifying suitable candidates efficiently
- Administrative automation reduces the paperwork burden
Implementation Example: A healthcare provider implemented AI-powered scheduling optimisation, reducing wait times by 41% while increasing facility utilisation by 27%, improving patient satisfaction and operational efficiency.
Professional Services
Transformative Applications:
- Intelligent document analysis extracting key information from contracts and reports
- Research assistance identifying relevant precedents and references
- Client service enhancement through personalised communication
- Project management optimisation predicting potential delays or issues
Implementation Example: A legal firm deployed AI-powered contract analysis, reducing review time by 63% while improving accuracy by identifying clauses missed in manual review. This significantly enhanced productivity and client service.
The Strategic Imperative of AI Adoption
Implementing artificial intelligence tools in business operations has evolved from experimental innovation to strategic necessity. As these technologies continue advancing, the competitive advantage gap between AI adopters and laggards will likely widen significantly.
Successful AI integration requires a thoughtful approach that combines clear business objectives, appropriate technology selection, strong data foundations, and organisational readiness. Businesses can build momentum and expertise before expanding to more complex applications by focusing initially on high-impact use cases with measurable outcomes.
While data quality, integration, skills, and governance challenges remain essential, they are increasingly manageable through structured implementation frameworks and growing ecosystem support. The potential benefits—enhanced efficiency, data-driven decision-making, personalised customer experiences, and competitive differentiation—substantially outweigh implementation hurdles.
For business leaders, the key question has shifted from whether to adopt AI to how quickly and effectively these technologies can be integrated into core operations. Organisations that develop comprehensive AI strategies aligned with business objectives, invest in necessary foundations, and commit to continuous learning and optimisation will be best positioned to thrive in an increasingly AI-powered business landscape.
Conclusion: AI for Business Success
As AI tools continue to reshape the business landscape, their impact on efficiency, decision-making, customer experience, and competitiveness is undeniable. The rapid adoption of AI technologies indicates that businesses across various sectors recognise the immense value these solutions offer. Whether it’s through enhancing operational efficiencies, delivering personalised customer experiences, or enabling data-driven decisions, AI is not just an option but an essential strategic asset. Organisations must focus on the careful and informed implementation of AI tools to ensure they derive maximum value while overcoming challenges like data security, integration with legacy systems, and skill gaps. Businesses that embrace AI will gain a competitive edge and set the stage for continuous innovation, enhanced scalability, and improved profitability.