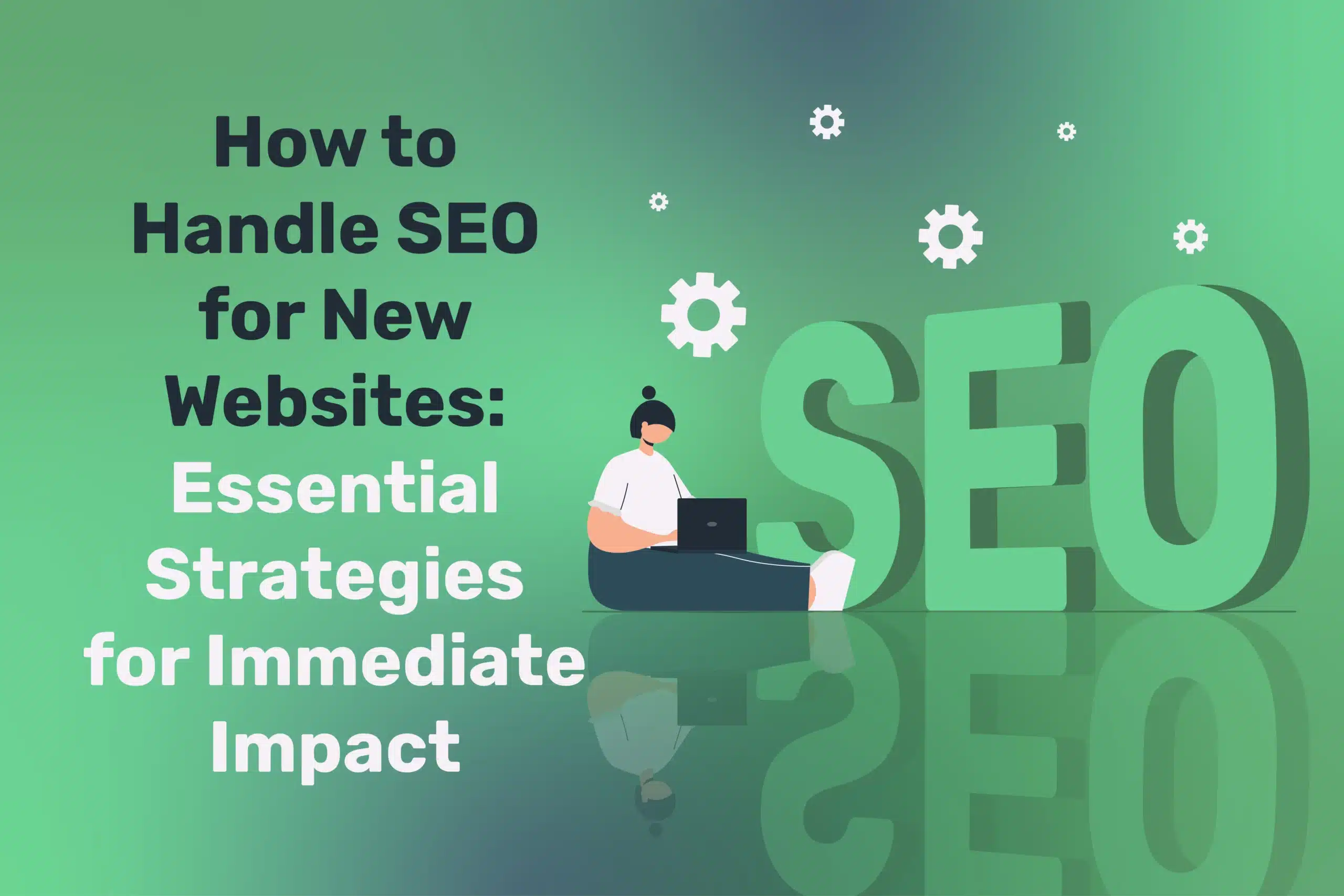
The Ethics of AI in Marketing: Transparency, Bias, and User Trust
Table of Contents
As AI drives personalisation, predictive recommendations, and automated ad targeting, many businesses and consumers are becoming increasingly concerned about privacy, algorithmic bias, and potentially manipulative marketing tactics. This comprehensive guide explores the ethical dimensions of AI in marketing—why transparency and user trust matter, the bias pitfalls that organisations must address, and how to remain a responsible brand in a data-driven world.
Why Ethics of AI in Marketing Are Crucial
Ethical AI marketing builds trust, ensures fairness, and prevents manipulation, fostering long-term customer relationships. As AI-driven strategies expand, maintaining transparency and accountability is essential for brand integrity and compliance.
Customer Backlash
If customers sense they’re being tracked or profiled without proper consent or transparency, brand reputation can rapidly deteriorate. Research shows that 86% of consumers are concerned about data privacy, with 79% unwilling to engage with brands they don’t trust with their data. This consumer awareness means ethical missteps can quickly escalate into viral social media criticism and lasting brand damage.
Regulatory Landscape
GDPR in Europe, CCPA in California, and other emerging data protection frameworks worldwide strictly limit how organisations can collect, process, and utilise personal data. Non-compliance penalties can be severe—up to €20 million or 4% of global annual turnover under GDPR. Ethical compliance isn’t just morally sound; it’s financially prudent and legally necessary.
“Ethical AI isn’t just about avoiding penalties or negative publicity,” says Ciaran Connolly, Director of ProfileTree. “It’s about building sustainable relationships with customers who increasingly expect brands to respect their privacy and use their data responsibly. The businesses that view ethics as a fundamental part of their strategy rather than a box-ticking exercise will be the ones that thrive in the long term.”
Trust as a Differentiator
In saturated markets where products and services are increasingly similar, how a brand handles customer data can become a powerful differentiator. Research indicates that 73% of consumers consider transparency about data usage “extremely important” when choosing which companies to support. Brands that handle data responsibly, clearly disclose usage practices, and avoid manipulative targeting build deeper customer loyalty and stronger market positions.
Common Ethical Concerns
Bias, data privacy, and transparency are key ethical concerns in AI marketing. Ensuring fairness, user consent, and responsible AI usage helps build trust and credibility.
Bias in Algorithms
AI systems reflect the data they’re trained on. If this training data excludes or underrepresents certain demographics, the resulting algorithms might produce discriminatory ad targeting, unfair pricing, or skewed content recommendations. These biases can perpetuate existing societal inequalities and damage brand reputation when discovered.
Examples of algorithmic bias in marketing include:
- Showing high-paying job adverts predominantly to male users
- Targeting credit card offers based on postcode areas that inadvertently discriminate against certain socioeconomic or ethnic groups
- Excluding older audiences from seeing certain product advertisements based on assumed preferences
Invasion of Privacy
Collecting overly granular personal data without clear consent can make customers feel their privacy is being violated. Practices such as cross-device tracking, browser fingerprinting, or using microphone access to inform advertising feel invasive to many consumers. A 2023 survey found that 67% of consumers have deleted apps or abandoned websites due to privacy concerns.
Manipulative Techniques
AI enables sophisticated psychological targeting that can exploit vulnerabilities. Concerning practices include:
- Dynamic pricing that charges more based on perceived willingness to pay
- Fear-based FOMO (fear of missing out) tactics that create artificial urgency
- Hyper-personalised emotional targeting that leverages known psychological triggers
- Addiction-forming design patterns that maximise engagement at the expense of user wellbeing
These approaches may boost short-term metrics but ultimately erode trust and can trigger regulatory scrutiny.
Identifying and Mitigating Bias
AI bias can lead to unfair targeting and misrepresentation in marketing. Regular audits, diverse training data, and transparency in algorithms help mitigate these risks.
Auditing Training Data
Organisations must thoroughly examine the data used to train marketing algorithms, recommendation engines, and targeting models. Key questions to ask include:
- Are certain demographic groups underrepresented or missing entirely?
- Does the data contain historical biases that might be perpetuated?
- Has the data been collected in ways that might systematically exclude certain populations?
- Are there gaps in the variables or attributes being measured?
Regular data audits should be a standard part of AI marketing implementation and maintenance.
Regular Performance Checks
Once deployed, AI marketing systems require continuous monitoring to ensure they don’t produce biased outcomes. Organisations should:
- Compare performance and recommendations across different customer segments
- Look for patterns in who receives particular offers or content
- Test systems with diverse user profiles to identify disparities
- Implement formal bias testing frameworks that examine racial, gender, age, and socioeconomic dimensions
Several specialist tools now exist specifically to highlight potential biases in marketing algorithms and advertising placement.
Inclusive Datasets
To prevent bias from the outset, organisations should:
- Actively seek diverse data sources that represent their entire customer base
- Supplement existing data with additional information from underrepresented groups
- Consider synthetic data generation to balance representation when necessary
- Partner with specialised agencies or consultants focused on inclusive data practices
This proactive approach ensures AI systems make fair and representative recommendations across all customer segments.
Transparency and Consent
Clear communication about data usage and obtaining user consent are essential for ethical AI marketing. Transparency fosters trust and ensures compliance with privacy regulations.
Clear Data Policies
Ethical AI marketing requires transparent communication about data practices. Best practices include:
- Plain-language privacy policies that avoid legal jargon
- Easily accessible summaries of how customer data influences marketing
- Visual explainers that illustrate data collection and usage
- Context-specific disclosures at relevant touchpoints
These approaches help customers make informed decisions about sharing their information.
Opt-Ins
For more sophisticated or sensitive data usage, explicit consent matters. Organisations should:
- Use clear, affirmative opt-in mechanisms rather than pre-ticked boxes
- Separate consent for different types of data usage rather than bundling them
- Make opting out as simple as opting in
- Provide granular options that allow customers to choose specific levels of personalisation
This approach respects customer autonomy and builds trust through transparent choice.
Explainable AI
When AI systems make decisions that affect customers, those decisions should be explainable. For example:
- “Products recommended based on your previous purchases and browsing history”
- “Discount offered based on your loyalty tier and purchase frequency”
- “Content selected based on interests you’ve indicated and similar customers’ preferences”
This transparency helps customers understand the “why” behind personalised marketing and builds confidence in the system’s fairness.
Designing Ethical AI Workflows
Building ethical AI workflows involves integrating fairness, accountability, and transparency at every stage. Ensuring bias mitigation, user privacy, and responsible data use are key to maintaining trust.
Human Oversight
AI systems should never operate completely autonomously in marketing contexts. Human oversight ensures that:
- Unusual patterns or potential biases are identified and addressed
- Edge cases receive appropriate handling
- Ethical considerations remain central to decision-making
- Systems function as intended without unexpected consequences
This hybrid approach combines AI efficiency with human judgement and ethical awareness.
User-Centric Goals
Marketing objectives should be framed in terms of genuine customer benefit rather than exploitation. Compare:
Exploitative approach: “Maximise time spent in app regardless of user benefit” Ethical approach: “Increase engagement by providing more relevant, valuable content”
Exploitative approach: “Target vulnerable users during emotional low points” Ethical approach: “Offer supportive content when it might be most helpful”
This customer-first mindset ensures marketing serves genuine needs rather than exploiting vulnerabilities.
Internal Review Committees
Formal ethics governance structures help organisations maintain high standards:
- Larger organisations might establish dedicated AI ethics committees
- Smaller businesses can implement regular ethical reviews of marketing practices
- Cross-functional teams should include perspectives from legal, marketing, data science, and customer service
- External advisors can provide objective assessment and specialised expertise
These governance mechanisms help identify potential issues before they affect customers.
The E-E-A-T Perspective on AI Ethics
Applying E-E-A-T to AI ethics means ensuring experience-driven, expert-informed, authoritative, and trustworthy AI practices. Transparency, accountability, and responsible data handling are essential for ethical AI implementation.
Experience
Demonstrate practical experience with ethical AI implementation:
- Document how your organisation has tested AI systems with diverse user groups
- Share case studies of how you’ve identified and addressed potential ethical issues
- Highlight your journey toward more ethical practices, including lessons learnt
- Showcase customer feedback that validates your ethical approach
This transparency builds credibility around your ethical commitments.
Expertise
Establish your organisation’s capabilities in ethical AI:
- Highlight relevant training or certifications held by your team
- Detail the methodologies you use to ensure ethical implementation
- Share thought leadership content on ethical marketing practices
- Explain how you stay current with evolving ethical standards and best practices
This expertise demonstrates your capacity to implement AI responsibly.
Authoritativeness
Reference established frameworks and standards:
- Cite recognised ethical guidelines such as the IEEE Ethically Aligned Design framework
- Mention industry-specific best practices or certification programmes
- Reference academic research that informs your approach
- Highlight partnerships with respected organisations focused on ethical AI
These external validations strengthen confidence in your ethical commitments.
Trustworthiness
Build trust through consistent ethical practices:
- Provide clear, accessible disclosures about data usage
- Offer meaningful user control over personal information
- Respond promptly and transparently to concerns or issues
- Demonstrate accountability when mistakes occur
- Show continuous improvement in your ethical practices
This accountability creates the foundation for lasting customer trust.
Case Example: Personalised Email Campaign
A personalised email campaign powered by AI can enhance user engagement, but ethical concerns like data privacy and bias must be addressed. Ensuring transparency and user consent helps maintain trust and align with E-E-A-T principles.
Scenario
An e-commerce brand implements an AI system that segments customers into “high spenders,” “bargain hunters,” and “infrequent buyers.” The system automatically sends tailored discount codes, product recommendations, and marketing messages based on these segments.
Ethical Pitfall
During a routine audit, the marketing team notices concerning patterns:
- The AI system disproportionately categorises older customers and those from certain postcode areas as “high risk,” never offering them the best promotions
- The logic appears to be making assumptions based on demographic factors rather than actual purchase behaviour
- This pattern creates potentially discriminatory outcomes that could harm both customers and the brand
Mitigation Strategy
The organisation takes several steps to address the issue:
- Immediately suspends the problematic segmentation rules
- Conducts a thorough review of the algorithm’s decision criteria
- Modifies the model to prioritise actual purchase behaviour rather than demographic proxies
- Implements ongoing monitoring specifically looking for similar bias patterns
- Updates customer communications to clearly explain the basis for personalised offers
- Creates an internal case study to ensure similar issues are prevented in future campaigns
This response demonstrates both ethical awareness and practical commitment to fair treatment.
Future Outlook: AI Ethics in Marketing
As AI continues to shape marketing strategies, ethical considerations will become even more critical. Future advancements must prioritise transparency, fairness, and consumer trust to ensure responsible AI-driven marketing practices.
Stricter Laws
The regulatory landscape continues to evolve rapidly:
- Future legislation may require organisations to demonstrate algorithmic fairness
- Consumers might gain expanded rights to explanation about automated decisions
- Marketing practices that appear manipulative face increasing regulatory scrutiny
- Penalties for non-compliance are likely to become more severe
Organisations that build ethical practices now will be better positioned for these changes.
Ethical AI Tools
The market for ethical AI solutions is expanding:
- Automated bias detection tools that integrate with marketing platforms
- Privacy-preserving analytics that reduce the need for individual-level data
- Federated learning approaches that keep personal data on user devices
- Explainable AI frameworks that make algorithm decisions more transparent
These tools make ethical implementation more accessible for organisations of all sizes.
Customer Demand
Consumer expectations around data ethics continue to rise:
- Customers increasingly seek clear explanations about how their data influences marketing
- Younger demographics in particular evaluate brands based on ethical data practices
- Transparency becomes a competitive advantage rather than simply a compliance requirement
- Brands that lag in ethical practices face growing consumer rejection
This shift makes ethical AI not just the right choice, but the commercially sound one.
Implementation Tips
Implementing ethical AI in marketing requires clear guidelines, continuous monitoring, and transparency. Prioritise user consent, minimise bias in data, and regularly audit AI-driven campaigns to ensure fairness and compliance.
Data Minimisation
Collect only the data you genuinely need:
- Audit existing data collection to identify unnecessary information
- Implement the principle of “data minimisation” across marketing activities
- Consider anonymised or aggregated approaches when individual-level data isn’t essential
- Regularly purge outdated or unnecessary personal information
This approach reduces both ethical risks and compliance burdens.
Regular Audits
Establish systematic review processes:
- Schedule regular audits of AI-based marketing systems
- Look specifically for evidence of bias or unintended consequences
- Test systems with diverse user profiles to identify potential issues
- Document findings and actions taken to address concerns
These reviews help catch problems before they affect customers or attract regulatory attention.
Customer Empowerment
Give customers meaningful control:
- Provide accessible preference centres that allow granular control over data usage
- Make opting out of specific marketing practices straightforward
- Offer clear explanations of how different settings affect the customer experience
- Create feedback mechanisms that allow customers to report concerns
This empowerment builds trust by demonstrating respect for customer autonomy.
Conclusion
AI offers marketing tremendous opportunities for personalisation and automation, but organisations must remain ethically vigilant. Transparent data usage, proactive bias identification, and respectful customer communication form the foundation of ethical AI marketing.
By acknowledging potential pitfalls—such as hidden bias or manipulative tactics—and addressing them through human oversight and systematic safeguards, organisations can reinforce brand integrity and cultivate genuine, long-term customer relationships.
In an environment increasingly shaped by data privacy concerns and growing consumer awareness, brand trust has become paramount. Ethical AI implementation isn’t merely a compliance exercise but a strategic imperative that delivers sustainable competitive advantage through stronger customer relationships and reduced regulatory risk.
ProfileTree specialises in helping businesses across Northern Ireland, Ireland, and the UK implement ethical AI solutions for marketing and customer engagement. Our team combines technical expertise with strategic insight to create systems that enhance both marketing effectiveness and ethical compliance. Contact us to discuss how we can help your business leverage AI responsibly while building customer trust and brand value.